How to implement Object Detection in Video with Gstreamer in Python using Tensorflow?
10 min. read |
In this tutorial we are going to implement Object Detection plugin for Gstreamer using pre-trained models from Tensorflow Models Zoo and inject it into Video Streaming Pipeline.
Requirements
- Ubuntu 18
- Python 3.6
- Gstreamer with Python Bindings. Look at “How to install Gstreamer Python Bindings on Ubuntu 18”
- gstreamer-python
- opencv-python
- tensorflow
- dvc
Code
Learn how to?
- Create gstreamer plugin that detects objects with tensorflow in each video frame using models from Tensorflow Models Zoo
Use gstreamer plugins
- sources: videotestsrc, filesrc
- transforms: videoconvert, decodebin, capsfilter
- sinks: fakesink, gtksink, autovideosink, fpsdisplaysink
Preface
In previous posts we’ve already learnt How to create simple Gstreamer Plugin in Python. Now let’s make a step forward.
Guide
Preparation
At, first clone repository with prepared models, video and code, so we can work with code samples from the beginning.
git clone https://github.com/jackersson/gst-plugins-tf.git
cd gst-plugins-tf
Use virtual environment to make dependencies for project clear and at own place. So, activate new one:
python3 -m venv venv
source venv/bin/activate
Then, install requirements from the project.
pip install --upgrade wheel pip setuptools
pip install --upgrade --requirement requirements.txt
For model inference install Tensorflow. But check if your PC supports Cuda-enabled GPU first (otherwise install CPU version):
# For just CPU support
pip install tensorflow==1.15
# For both CPU | GPU support
pip install tensorflow-gpu==1.15
Additional. To make projects reproducible at any time I prefer to use Data Version Control for models, data or other huge files. As a storage service I use Google Cloud Storage (free, easy to use and setup).
export GOOGLE_APPLICATION_CREDENTIALS=$PWD/credentials/gs_viewer.json
dvc pull
Now check data/ folder there should be prepared model (.pb) and video (.mp4), so you can easily run tests on your own.
Define baseline gstreamer pipeline
Launch next pipeline in terminal to check that gstreamer works properly
gst-launch-1.0 filesrc location=data/videos/trailer.mp4 ! decodebin ! \
videoconvert ! video/x-raw,format=RGB ! videoconvert ! autovideosink
Basically, the following pipeline :
- captures frames from video file usig filesrc,
- converts frames to RGB colorspace using videoconvert and capsfilter with pre-defined image colorspace format by a string “video/x-raw,format=RGB“.
- displays frames in window with autovideosink
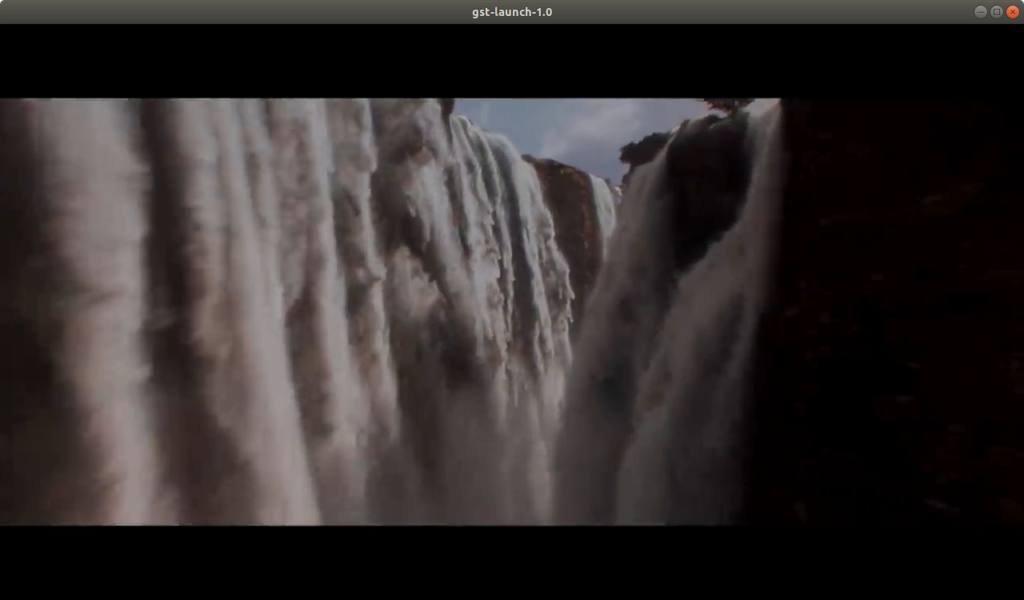
Run
Display mode
For now, let’s run a simple predefined command to check that everything working:
./run_example.sh
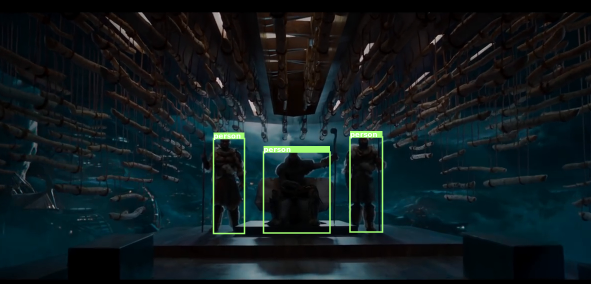
Text mode
Export required paths to enable plugin and make it visible to gstreamer:
export GST_PLUGIN_PATH=$GST_PLUGIN_PATH:$PWD/venv/lib/gstreamer-1.0/:$PWD/gst/
Note
$PWD/venv/lib/gstreamer-1.0/
- Path to libgstpython.cpython-36m-x86_64-linux-gnu.so (built from gst-python)
$PWD/gst/
- Path to Gstreamer Plugins implementation (python scripts)
Run command with enabled debug messages print:
Note: check gstreamer debugging tools to enable logging
GST_DEBUG=python:5 \
gst-launch-1.0 filesrc location=data/videos/trailer.mp4 ! \
decodebin ! videoconvert ! \
gst_tf_detection config=data/tf_object_api_cfg.yml ! \
videoconvert ! fakesink
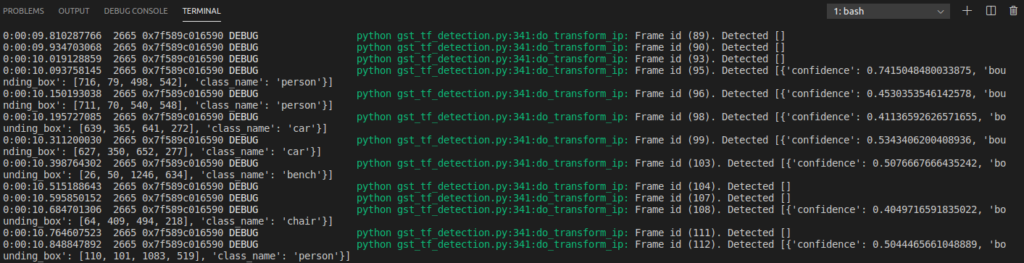
Note: application should print similar output (list of dicts with object’s class_name, confidence, bounding_box)
[{'confidence': 0.6499642729759216, 'bounding_box': [402, 112, 300, 429], 'class_name': 'giraffe'}, \
{'confidence': 0.4659585952758789, 'bounding_box': [761, 544, 67, 79], 'class_name': 'person'}]
Great, now let’s go through code.
Explanation
From previous post (ex.: How to write a Gstreamer Plugin with Python) we discovered that from gstreamer plugin we can easily get image data. Now let’s try to run model on retrieved image data and display inference results in console or video.
At the beginning let implement Object Detection Plugin (gst_tf_detection).
Define plugin class
First, define Plugin class that extends GstBase.BaseTransform (base class for elements that process data). Plugin’s name “gst_tf_detection” (with this name plugin can be called inside gstreamer pipeline).
class GstTfDetectionPluginPy(GstBase.BaseTransform):
GST_PLUGIN_NAME = 'gst_tf_detection'
Fixate stream format
First, define input and output buffer’s format for plugin. Since our models consumes RGB image data, let’s specify it:
_srctemplate = Gst.PadTemplate.new('src', Gst.PadDirection.SRC, Gst.PadPresence.ALWAYS, Gst.Caps.from_string("video/x-raw,format=RGB"))
_sinktemplate = Gst.PadTemplate.new('sink', Gst.PadDirection.SINK, Gst.PadPresence.ALWAYS, Gst.Caps.from_string("video/x-raw,format=RGB"))
Define properties
Additionally, let define next parameters to be able to pass:
- model instance (with proper interface).
- to be able to pass python object use GObject.TYPE_PYOBJECT as parameter type
- model config, so we can easily modify model’s parameters without changing single line of code.
- to be able to pass string use str as parameter type.
- configuration file with parameters is common practice to setup plugins when the number of parameters exceeds 3 and more.
__gproperties__ = {
"model": (GObject.TYPE_PYOBJECT,
"model",
"Contains Python Model Instance",
GObject.ParamFlags.READWRITE),
"config": (str,
"Path to config file",
"Contains path to config file supported by Python Model Instance",
None, # default
GObject.ParamFlags.READWRITE
),
}
Note: Passing model as a parameter also allows to save memory consumption. For example, if model is initialized each time plugin created then model’s weights are duplicated in the memory. In a such way the number of running pipelines simultaneously is limited by memory amount. But if model is created once and passes to each plugin as a reference, then the only limitation is hardware performance capacity.
Now, to specify model’s config use next command
gst_tf_detection config=tf_object_api_cfg.yml
Implement get-set handlers for defined properties
GET
def do_get_property(self, prop: GObject.GParamSpec):
if prop.name == 'model':
return self.model
if prop.name == 'config':
return self.config
else:
raise AttributeError('Unknown property %s' % prop.name)
SET
def do_set_property(self, prop: GObject.GParamSpec, value):
if prop.name == 'model':
self._do_set_model(value)
elif prop.name == "config":
self._do_set_model(from_config_file(value))
self.config = value
Gst.info(f"Model's config updated from {self.config}")
else:
raise AttributeError('Unknown property %s' % prop.name)
When config for model is updated we need to shutdown previous one, initialize and start new one.
def _do_set_model(self, model):
# stop previous instance
if self.model:
self.model.shutdown()
self.model = None
self.model = model
# start new instance
if self.model:
self.model.startup()
Implement transform()
First, define a function to do buffer processing in-place do_transform_ip(), that accepts Gst.Buffer and returns state (Gst.FlowReturn).
def do_transform_ip(self, buffer: Gst.Buffer) -> Gst.FlowReturn:
Then, if there is no model plugin should work in passthrough mode.
if self.model is None:
Gst.warning(f"No model speficied for {self}. Plugin working in passthrough mode")
return Gst.FlowReturn.OK
Otherwise, we convert Gst.Buffer to np.ndarray, feed image to model (inference), print results to console and write objects to Gst.Buffer as metadata (recap: How to add metadata to gstreamer buffer), so detected objects can be transmitted further in pipeline.
import gstreamer.utils as utils
from gstreamer.gst_objects_info_meta import gst_meta_write
# Convert Gst.Buffer to np.ndarray
caps = self.sinkpad.get_current_caps()
image = utils.gst_buffer_with_caps_to_ndarray(buffer, caps)
# model inference
objects = self.model.process_single(image)
Gst.debug(f"Frame id ({buffer.pts // buffer.duration}). Detected {str(objects)}")
# write objects to as Gst.Buffer's metadata
gst_meta_write(buffer, objects)
Tensorflow Model Implementation
We won’t deep dive much into Tensorflow model implementation. Just have a look at code. Class TfObjectDetectionModel hides:
- tf.Graph import
- device configuration
- model parameters (ex.: threshold, labels, input size)
Additional
Model Configuration file
- file with common editable parameters for model inference. For example:
weights: "model.pb"
width: 300
height: 300
threshold: 0.4
device: "GPU|CPU"
labels: "labels.yml"
log_device_placement: false
per_process_gpu_memory_fraction: 0.0
Labels format
- file with lines of pairs <class_id: class_name>. For example:
1: person
2: bicycle
3: car
4: motorcycle
...
90: toothbrush
Object Detection Overlay Plugin
In order to draw detected objects on video there is an implementation of gst_detection_overlay plugin (recap: “How to draw kitten with Gstreamer“).
Main differences compared to gst_tf_detection plugin.
Input-output buffer’s format now RGBx (4-channels format), so we can work with buffer using cairo library.
Gst.Caps.from_string("video/x-raw,format={RGBx}")
Request detected objects info from buffer using gstreamer-python package
from gstreamer.gst_objects_info_meta import gst_meta_get
objects = gst_meta_get(buffer)
To enable drawing on buffer (in-place) use gstreamer-python package as well
from gstreamer import map_gst_buffer
import gstreamer.utils as utils
caps = self.sinkpad.get_current_caps()
width, height = utils.get_buffer_size_from_gst_caps(caps)
# Do drawing
with map_gst_buffer(buffer, Gst.MapFlags.READ | Gst.MapFlags.WRITE) as mapped:
draw(mapped, width, height, objects)
Tuning
- change model
- Now you can download any Object Detection model from Tensorflow Models Zoo
weights: "path/to/new/model/frozen_inference_graph.pb"
- change video input
- run whole pipeline on your video file, from camera or stream
- change model’s config
- reduce false positives with higher confidence threshold
threshold: 0.7
- improve quality with increasing input size
width: 600
height: 600
- leave target labels only
1: person
3: car
Conclusion
With Gstreamer Python Bindings you can inject any Tensorflow model in any video streaming pipeline. Custom plugins with Tensorflow models already used by popular video analytics frameworks.
Hope everything works as expected 😉 In case of troubles with running code leave comments or open an issue on Github.
No element “gst_tf_detection”
I ran into this error when i tried to implement the example. U have any idea can help me ?Thanks alot
Hi,
Here I tried it on another PC:
—————-
git clone https://github.com/jackersson/gst-plugins-tf.git
#
# Create environment
#
cd gst-plugins-tf/
python3 -m venv venv
source venv/bin/activate
pip install -r requirements.txt
#
# Make plugins visible
#
export GST_PLUGIN_PATH=$PWD/gst # Fixed path in repository
#
# create symbol link for gi package
#
ln -s /usr/lib/python3/dist-packages/gi venv/lib/python3.6/site-packages
#
# Check plugin works
#
gst-inspect-1.0 gst_tf_detection
> Prints plugin's info
To get error message (Not just "No element “gst_tf_detection”":
rm -rf ~/.cache/gstreamer-1.0
and then:
gst-inspect-1.0 gst_tf_detection
——————-
Hope this works)
To fix this I needed to install libgst_objects_info_meta.so from your https://github.com/jackersson/pygst-utils into venv/lib/python3.6/site-packages/pygst_utils/3rd_party/gstreamer/build/.
Hi, Jose
Thanks for update.
Just checked that this dependency is present in requirements.txt. Next commands should help you to avoid described problem.
python3 -m venv venv
source venv/bin/activate
pip install -r requirements.txt
Best regards,
Taras Lishchenko
I have a similar problem. I got
“OSError: /home/pavel/Workout/PluginUploadVenv/lib/python3.6/site-packages/pygst_utils/3rd_party/gstreamer/build/libgst_objects_info_meta.so: cannot open shared object file: No such file or directory”
I did not find libgst_objects_meta_info anywhere, installing with requirements did not help. Help me please!
PS: i m stupid in gstreamer, do not blame)
I am having a similar problem as the above poster- I am working on a Jetson TX2 and am receiving the following error message when I try to run the example plugin (after removing ~/.cache/gstreamer-1.0):
(gst-plugin-scanner:25395): GStreamer-WARNING **: Failed to load plugin ‘/usr/lib/aarch64-linux-gnu/gstreamer-1.0/libgstclutter-3.0.so’: /usr/lib/aarch64-linux-gnu/libgbm.so.1: undefined symbol: drmGetDevice2
WARNING: erroneous pipeline: no element “gst_tf_detection”
Any help would really be appreciated!
Hi, Caroline McKee
Thank you for your question.
This seems to be OpenGL problem. Can you share the pipeline itself, that you are using? (Maybe you are using some OpenGL plugins for decoding/visualization)
Make sure that you are using proper pipeline On Jetson TX2. For example:
gst-launch-1.0 filesrc location=video.mp4 ! qtdemux name=demuxer_0 demuxer_0.video_0 ! h264parse ! omxh264dec ! nvvidconv ! video/x-raw,format=RGBA ! videoconvert n-threads=0 ! video/x-raw,format=RGB ! gst_detection_tf ! fpsdisplaysink video-sink=fakesink sync=false signal-fps-measurements=True
Best regards,
Taras Lishchenko
I am having a very similar problem to the above commenter. I am working on a Jetson TX2 and when I try to run your example plugin, I get the follow error message (after removing ~/.cache/gstreamer-1.0):
(gst-plugin-scanner:25395): GStreamer-WARNING **: Failed to load plugin ‘/usr/lib/aarch64-linux-gnu/gstreamer-1.0/libgstclutter-3.0.so’: /usr/lib/aarch64-linux-gnu/libgbm.so.1: undefined symbol: drmGetDevice2
WARNING: erroneous pipeline: no element “gst_tf_detection”
Any help would be really appreciated!
The first and second warning seem to be unrelated.
I’m not sure about the first issue, But the second issue is most likely due to Gstreamer on the Jeston is missing the Python binding: https://github.com/GStreamer/gst-python.
Unfortunately, compiling Gst-Python on the Nano was not an easy task due to a series of dependencies and bugs. In my case I ended up building:
OpenCV 4.0 with Cuda enabled (in the Jetson Nano it’s not enabled out of the box, but I don’t clearly remember if this was a dependency, so you might be able to skip this one)
GCC 7.4, manually patched with https://gcc.gnu.org/bugzilla/show_bug.cgi?id=89752, otherwise Tensorflow won’t compile.
Tensorflow 1.13.1 with the above GCC (including its Python Wheel)
Then GST-Python.
gst_tf detection issue
DRM_IOCTL_I915_GEM_APERTURE failed: Invalid argument
Assuming 131072kB available aperture size.
May lead to reduced performance or incorrect rendering.
get chip id failed: -1 [22]
param: 4, val: 0
DRM_IOCTL_I915_GEM_APERTURE failed: Invalid argument
Assuming 131072kB available aperture size.
May lead to reduced performance or incorrect rendering.
get chip id failed: -1 [22]
param: 4, val: 0
DRM_IOCTL_I915_GEM_APERTURE failed: Invalid argument
Assuming 131072kB available aperture size.
May lead to reduced performance or incorrect rendering.
get chip id failed: -1 [22]
param: 4, val: 0
No such element or plugin ‘gst_tf_detection
file inside gst-plugins/gst/python/gst_tf_detection.py
DRM_IOCTL_I915_GEM_APERTURE failed: Invalid argument
Assuming 131072kB available aperture size.
May lead to reduced performance or incorrect rendering.
get chip id failed: -1 [22]
param: 4, val: 0
DRM_IOCTL_I915_GEM_APERTURE failed: Invalid argument
Assuming 131072kB available aperture size.
May lead to reduced performance or incorrect rendering.
get chip id failed: -1 [22]
param: 4, val: 0
DRM_IOCTL_I915_GEM_APERTURE failed: Invalid argument
Assuming 131072kB available aperture size.
May lead to reduced performance or incorrect rendering.
get chip id failed: -1 [22]
param: 4, val: 0
No such element or plugin ‘gst_tf_detection
Very good work
Just I wanted to share with you another way to run_example.sh directly from you-tube
assuming you install youtube-dl
refering to another post you made
http://lifestyletransfer.com/how-to-watch-youtube-videos-with-gstreamer/
(venv) root@debian-c1:~/work/py/tf/gst-plugins-tf# cat run_youtube.sh
#!/bin/bash
export TF_CPP_MIN_LOG_LEVEL=5
export GST_PLUGIN_PATH=$GST_PLUGIN_PATH:$PWD/venv/lib/gstreamer-1.0/:$PWD/gst/
export GST_DEBUG=python:4
file=$1
[ -z “$file” ] && file=data/videos/trailer.mp4
#gst-launch-1.0 filesrc location=${file} ! decodebin ! videoconvert ! gst_tf_detection config=data/tf_object_api_cfg.yml ! videoconvert ! gst_detection_overlay ! videoconvert ! autovideosink
gst-launch-1.0 souphttpsrc is-live=true location=”$(youtube-dl –format “best[ext=mp4][protocol=https]” –get-url ${file})” ! decodebin ! videoconvert ! gst_tf_detection config=data/tf_object_api_cfg.yml ! videoconvert ! gst_detection_overlay ! videoconvert ! autovideosink
Great, Eric 😉 Thank you!
Hi,
Great tutorial, thank you!
I wanted to adapt your example in order to store the meta-data of a bounding box in each frame, which is then livestreamed as h264 over then network via udpsrc/sink. However, it seems the metadata get lost in the transmission udpsink->udpsrc. The following “pipeline” works fine: raw/h264 ! write-meta-data-to-buffer-plugin ! x264enc ! rtph264pay ! rpth264depay ! decode ! draw-meta-data ! autovideosink.
If I split this pipeline with dupsrc/sink like:
sender: …. ! rtph264pay ! udpsink port=xxxx
reciever: udpsrc port=xxxx ! rpth264depay ! decode ! ….
the obtained meta-data is empty:/ The video-streaming works perfectly though..
Did you ever try/manage to send the meta-data via udpsink/src?
Hi Remmius,
Unfortunately, metadata transmission is not quite supported. As I understand Gstreamer supports video, audio, text(subtitles) for encoding/decoding. And in order to pass own meta-data you should create own encoder/decoder.
For example, when you break pipeline with udpsink the only data that sends to udpsrc is H264 encoded stream, that contains only video data.
The reason your single pipeline works is that in Gstreamer pipeline plugins exchange data as Gst.Buffer, that can store metadata separately from image data. But Gst.Buffer is not going to be sent via network.
If you come up with any simple solution, – let me know 😉
Best regards,
Taras,
Excellent tutorial! Very helpful.
Could you briefly comment on what would need to be tweaked in your gst_tf_detection.py code to allow for a plugin that can handle arbitrary tensorflow frozen graph models (not just detection models). In particular, how would you do head pose estimation with something like https://github.com/shamangary/FSA-Net ?
Thanks,
Rob
Hi Robert,
Thank you 😉
I was planning to do similar task (pose estimation). But in general, to make gstreamer handle any model I propose the next steps:
1. Implement model inference class (similar to TfObjectDetectionModel). It could be TF, Keras, Pytorch. The key is to define method: process_single(np.ndarray)
2. Define model config (similar to one in TfObjectDetectionModel). This allows you to tweak parameters without modifying any code.
3. Implement Gstreamer Plugin (but in general GstTfDetectionPluginPy doesn’t depend on model implementation).
Those are the base steps.
Additional step:
1. Custom metadata (Only if there is a plan to pass detections further in gstreamer pipeline). Use the following guide “How to add metadata to gstreamer buffer”
Also I suggest to look at approach when you capture buffers from gstreamer pipeline using appsink (tutorial). This approach is much easier and more Pythonic 😉
Hope this helps 😉
Best regards,
Taras
Taras,
I can run the CLI gstreamer pipelines just fine, but when I build the pipelines inside a python app, the gst_tf_detection and gst_detect_overlay seem to be completely ignored. For example, when I build the pipeline as
self.player = Gst.Pipeline.new(“player”)
source = Gst.ElementFactory.make(“v4l2src”, “usb-cam-source”)
source.set_property(‘device’, “/dev/video0”)
vidconv = Gst.ElementFactory.make(‘videoconvert’)
caps = Gst.Caps.from_string(“video/x-raw,format=RGB,width=640,height=480”)
capsfilt = Gst.ElementFactory.make(‘capsfilter’,’filter’)
capsfilt.set_property(“caps”, caps)
tf_detect = Gst.ElementFactory.make(‘gst_tf_detection’,’tf_detect’)
tf_detect.set_property(‘config’, “path/to/tf_object_api_cfg.yml”)
vidconv2 = Gst.ElementFactory.make(‘videoconvert’)
detect_overlay = Gst.ElementFactory.make(‘gst_detection_overlay’,’detect_overlay’)
vidconv3 = Gst.ElementFactory.make(‘videoconvert’)
sink = Gst.ElementFactory.make(‘autovideosink’)
sink.set_property(‘sync’, “False”)
# Add elements to pipeline
self.player.add(source)
self.player.add(vidconv)
self.player.add(capsfilt)
self.player.add(tf_detect)
self.player.add(vidconv2)
self.player.add(detect_overlay)
self.player.add(vidconv3)
self.player.add(sink)
# Link everything together
source.link(vidconv)
vidconv.link(capsfilt)
capsfilt.link(tf_detect)
tf_detect.link(vidconv2)
vidconv2.link(detect_overlay)
detect_overlay.link(vidconv3)
vidconv3.link(sink)
inside my app and run it, there are no errors but also no object detection being performed; only the unannotated source video plays out. Any ideas?
Rob
Hi,
I suggest you to use Gst.parse_launch instead. With this approach there will be no problems.
Have a similar problem when connecting everything manually (like you provided in example). But same problem occurs even when using plugins from examples (gst-python). It seems some base class (GstBase.BaseTransform) functions not initialized properly or not overridden by child class (GstTFPluginPy)
Best regards,
Taras
Thanks, Taras!
Using Gst.parse_launch fixed the problem for running static pipelines. However, in my application the pipeline is dynamic and is built incrementally and conditionally using the “pad-added” signal and with function callbacks. If you find a way to make your gst_tf_detection element work via the python pipeline “build-add-link” approach I was trying to use, please let me know!! I’m desperate to try to get TF plugin capability working in my app. ;}
Thanks again,
Rob
Hi,
I’ll go back to this question in a few days. Meanwhile you can check another approach: register gstreamer plugin only for particular application.
For example:
1. Plugin implementation: gstplugin_py
2. Plugin registration for application example . Just call the following before element creation.
register(GstPluginPy)
I used this approach successfully for some of my projects. And it worked with creating pipeline with Gstreamer Element Factory 😉
I’ll try to do tutorial on this in a week and share with you 😉
Feel free to keep me updated with any news on your project progress.
Best regards,
Taras
Hi,
As mentioned in Readme, – try to use the next export:
export GST_PLUGIN_PATH=$GST_PLUGIN_PATH:$PWD/venv/lib/gstreamer-1.0/:$PWD/gst/
where:
$GST_PLUGIN_PATH – previous plugins path
$PWD/venv/lib/gstreamer-1.0 – path to libgstpython.cpython-36m-x86_64-linux-gnu.so
$PWD/gst/ – path to python/ folder with *.py plugins implementations (in your case: /home/sai/gst-plugins-tf/gst/)
Also sometimes cleaning gstreamer cache helps:
rm -rf ~/.cache/gstreamer-1.0/
Hope this helps 😉
Best regards,
Taras
Hi dear,
Thanks a lot for sharing knowledge about gstreamer.
I want to know in your opinion, Isn’t there a problem for writing the custom gstreamer plugins in python on device in production. constrained device? Have you experience working for writing custom gstreamer plugin in c++ and python? How much the C++ has performance than python?
Hi Ayhan,
I work mostly with image processing tasks, so my decision making algorithm is the following:
1. Use OpenCV (instead of Gstreamer) if sufficient for video capturing (Python, PoC). Mostly for cases: single video stream, no timing-synchronization, video recording, …
2. Use Gstreamer (Python), when not (1). Mostly it’s connected to: multiple video processing pipelines, usage of existing pre-post processing plugins or hardware-accelerated, visualization of metadata (cute video overlays), video recording, IOT-platforms.
3. Use Gstreamer (C++), when: working with capture device drivers, memory management (allocate buffer, GPU memory layouts). Mostly: use C++ when Python is not capable.
Note: Also if you use inside Python plugin any C/C++ based libs (numpy, opencv, tensorflow, …) then in most cases there is no performance drop.
Note 2: In most my commercial projects for Video Surveillance we used Gstreamer (both Python and C++-when-Python-just-not-capable). The only plugins I wrote in C: Video Source for Intel Realsense Camera (in 2016), QR-Code detection for Robot platform (in 2019). In most other cases Python was sufficient.
P.S. I am myself inspired by DeepStream SDK, DLStreamer
Hope this helps 🙂
Thanks
I want to create a custom gstreamer buffer and added to deepstream.
My goal is to create inference plugin gstreamer like face recognition plugin and add to deepstream pipeline.
This work has two Question:
I want to use python in do_transform_ip() method:
1- How to pass gst-buffer to inferecne model? I don’t want to convert buffer to numpy array.
2- Rescale the buffer before feed to model.
Has a converting buffer to numpy array overload? specially in jetson devices that have share memory.